Berkeley Earth is dedicated to advancing the field of climate science by providing open-source, high-resolution datasets that enhance the understanding of local climate dynamics and risks. In keeping with this ethos, today we introduce our forthcoming Climate Model Synthesis project, which aims to address critical gaps in existing climate models by applying bias correction and downscaling techniques to produce more accurate, locally relevant projections. This work represents a significant step forward in the field of climate data science, and will mark the production of Berkeley Earth’s first forward-looking data product. We expect this new product will provide unprecedented access to actionable local climate insights, thereby helping to inform adaptation and mitigation strategies for the changing planet.
Below we provide an introduction to the motivation behind this project, as well as preview a sample of initial results.
In advance of the full release, we welcome expressions of interest from potential partners interested in being among the first to work with these new products. An initial data set is expected in Q1 of 2025, with publication to follow later next year.
Climate Models have performed very well when predicting overall warming trends at global scales…
Despite facing frequent criticism, global climate models (GCMs), specifically those that make up the CMIP6 collection of models, have exhibited skill at recreating the warming trend observed in the global average temperature record. Given this performance modeling historic trends, there is generally confidence in the ability of global climate models to predict future global average temperatures, including when the world might cross the 1.5°C threshold defined by the Paris Agreement.

Figure 1: While there is some evidence suggesting that the rate of global warming has increased in recent decades, recent warming is largely consistent with CMIP6 model projections. In an October 2024 analysis originally published in the Climate Brink, Berkeley Earth’s Zeke Hausfather found that 2023’s exceptionally warm temperatures remained broadly consistent with CMIP6 climate model projections. The figure at left, taken from this analysis, reflects monthly global surface temperature anomalies from CMIP6 climate models (using SSP2-4.5) and observations between 1970 and 2030 using a 1900-2000 baseline period.
…but these same climate models tend to over-or underestimate warming when downscaled for projections at city and local scales.
Global climate models were designed and calibrated to reflect trends in climatology on large scales; however, modeled projections have a greater tendency to diverge from observations in downscaled, local modeling contexts, presenting challenges and questions about how to best leverage global climate models to predict future climate states at local scales.

Figure 2: Local climate trends depend more heavily on weather patterns, topography, and other local nuances that tend to be masked when averaged out across the entire planet. Here, the observed warming trend in Miami, Florida is higher than predicted by nearly all of the 44 CMIP6 global climate models, suggesting that the raw output of these GCMs, including the model mean, has historically underestimated local warming rates in Miami, calling into question their ability to accurately represent future warming.
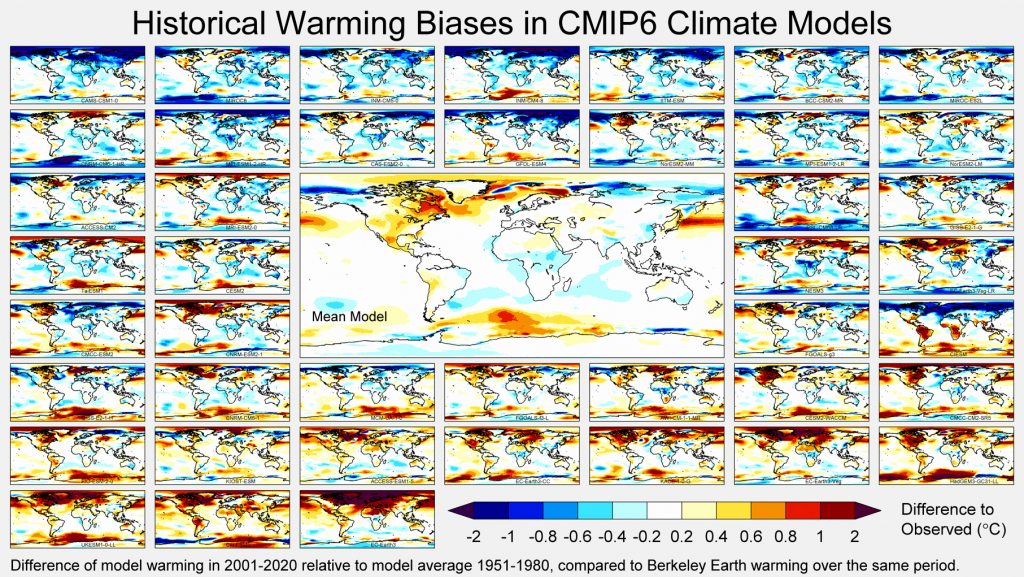
Figure 3: Averaging all models equally is unlikely to be the best way of reducing model error. During the last IPCC cycle, a subset of climate models showed rates of warming too large to be consistent with historical and paleoclimate observations. For the first time, this “hot models” problem led the IPCC to abandon true model democracy in favor of selecting and weighting models based on a single globally-averaged metric, the climate sensitivity. Unfortunately, this approach still considers only a very limited view of model accuracy, and fails to consider observed regional variations in model performance. We can do better.
By identifying and correcting systematic differences between models and observations, we can significantly improve the accuracy of climate models in downscaled scenarios.
Climate models often struggle to accurately reproduce features at the intersection between weather and climate, such as annual record high temperatures or estimates of cooling degree days. Such metrics requires the model to accurately reproduce the climatology, seasonality, and daily variability of the weather in a specific location.
Figure 4: Berkeley Earth’s Climate Model Synthesis represents a bias-corrected and downscaled surface temperature data product synthesized from 40 GCMs and 363 variants spanning five shared socioeconomic pathways. Bias correction is achieved by comparing historical simulations to ERA5 and Berkeley Earth’s new high resolution dataset.

Figure 5: After decomposing surface temperature from each grid cell to adjust for long-term climatology, seasonality, and weather variability, an overall synthesis is produced by training a basic weather emulator on the biased corrected models and using historical accuracy as a guide to model selection and weight. This allows us produce hundreds of simulated scenarios that span the variability across the models, including daily extremes.
After bias-correcting against historical observations, the model spread & implied uncertainty at 2100 is greatly reduced, often by ~50%.

This bias correction creates more accurate, more actionable projections of future climate states downscaled to city-level
By integrating outputs from multiple models, weighted according to their regional accuracy, and applying machine learning techniques for downscaling, the synthesis will provide more reliable, high-resolution projections that accurately reflect local climate variations. This methodology reduces uncertainties associated with traditional ensemble methods, offering stakeholders more precise data for assessing localized climate risks and making informed adaptation decisions.

Figure 6: Projected annual maximum temperatures in Dallas across four decades comparing raw climate model outputs from the CMIP6 SSP2-4.5 ensemble with bias-corrected data. The raw model projections (left) exhibit considerable variability, and tend to overestimate future temperature extremes. After bias correction (right), the probabilities becomes more consistent and accurate, with the corrected projections reflecting a range of temperatures that better matches observed historical patterns. This improved accuracy provides a clearer and more realistic prediction of extreme temperature events, helping to refine local adaptation strategies and resilience planning.

Figure 7: From the bias-adjusted data set we can begin extracting useful, relevant data sets to help inform future adaptation planning. Cooling Degree Days (CDD) are a vital metric for understanding the demand for cooling in a specific location, quantifying how much cooling is needed based on daily temperature variations. By applying bias correction to CDD projections, we can provide more accurate and reliable forecasts of future cooling needs, ensuring that local risk models account for realistic temperature extremes. This refined data helps businesses and communities better prepare for and respond to the growing demand for cooling due to rising temperatures.
Next Steps / Coming soon
As we continue to enhance our suite of open-source climate data tools, we are focused on making The Climate Model Synthesis data set even more accessible and impactful for local communities, businesses, and policymakers. Here’s how we plan to build on this critical work:
- A data set of future temperature projections, including TAVG, TMAX, TMIN, and related metrics, is expected by Q1 2025
- A corresponding precipitation data set, including extremes both high and low, is expected in late 2025
- Additional variables for development under consideration include wind, hail, storminess, etc.
- Berkeley Earth is also currently developing additional products to support rapid attribution and more effective characterization of extreme events. More information will be available in early 2025.

Join Us
We invite you to express your interest in joining us on this groundbreaking initiative to advance climate science through more accurate and accessible data tools. By participating, you will contribute to the development of cutting-edge solutions that empower local communities, policymakers and other key stakeholders in mitigating and adapting to the impacts of climate change.
This project is made possible through the generous support of Quadrature Climate Foundation and the Patrick J. McGovern Foundation. Their commitment to advancing climate science and data-driven solutions is instrumental in enabling Berkeley Earth to develop critical tools for local climate resilience and adaptation.